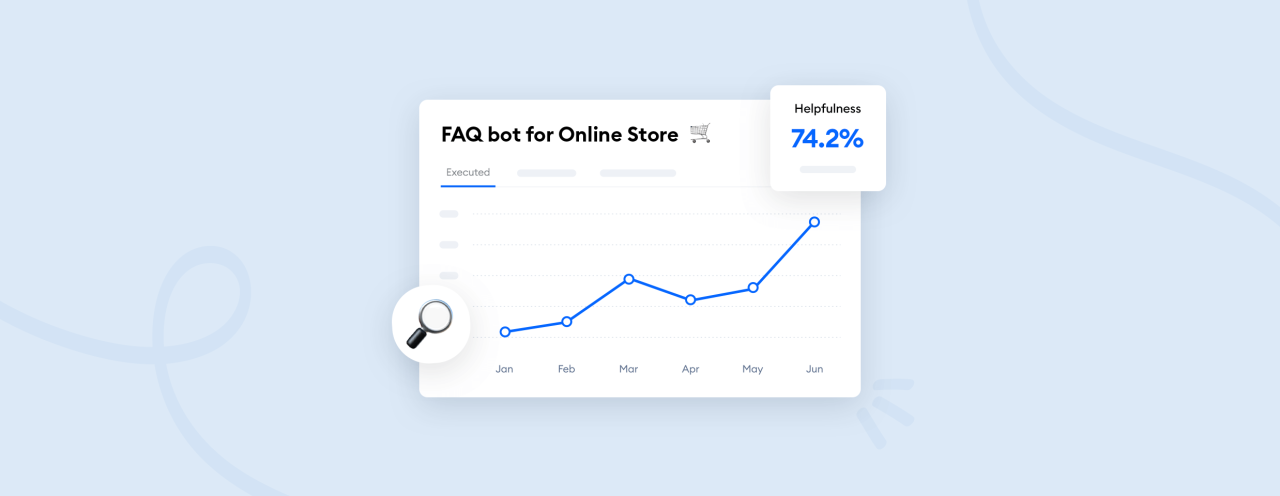
Ai Chatbot Performance Metrics
AI chatbot performance metrics set the stage for this enthralling narrative, offering readers a glimpse into a story that is rich in detail and brimming with originality from the outset. As we delve into the world of chatbots, these metrics provide invaluable insights into optimizing their performance and enhancing user experiences.
Exploring the different types of metrics, from user engagement to conversational quality, opens up a world of possibilities for chatbot developers and businesses looking to leverage this technology effectively. Let’s dive deeper into the realm of AI chatbot performance metrics and discover the keys to success in this evolving landscape.
Definition of AI Chatbot Performance Metrics
AI chatbot performance metrics are quantitative measures used to evaluate the effectiveness and efficiency of a chatbot in interacting with users. These metrics help businesses assess how well their chatbots are performing in terms of customer satisfaction, response time, accuracy, and overall user experience.
Examples of Common Metrics Used to Evaluate Chatbot Performance
- Response Time: The time it takes for the chatbot to respond to a user query.
- Accuracy: The percentage of correct responses provided by the chatbot.
- Engagement Rate: The level of user interaction and engagement with the chatbot.
- Retention Rate: The percentage of users who continue to use the chatbot over time.
- Resolution Rate: The rate at which the chatbot successfully resolves user issues or queries.
Tracking these metrics allows businesses to identify areas for improvement and optimize their chatbots for better performance.
Types of AI chatbot performance metrics
AI chatbot performance metrics can be categorized into qualitative and quantitative metrics, each providing valuable insights into the effectiveness of chatbots in various aspects. Let’s explore the different types of metrics used to measure chatbot performance.
Qualitative performance metrics, AI chatbot performance metrics
Qualitative performance metrics focus on the quality of interactions and user experience provided by chatbots. These metrics are subjective in nature and are often obtained through feedback and observation. Some examples of qualitative performance metrics include:
- Conversation quality: Evaluating the natural flow and coherence of conversations between the chatbot and users.
- User satisfaction: Measuring the level of satisfaction users experience when interacting with the chatbot.
- Sentiment analysis: Analyzing the emotions and sentiments expressed by users during interactions with the chatbot.
Quantitative performance metrics
Quantitative performance metrics, on the other hand, focus on measurable data and statistics to assess the performance of chatbots. These metrics provide concrete numbers that can be analyzed to identify areas for improvement. Some examples of quantitative performance metrics include:
- Response time: Calculating the average time taken by the chatbot to respond to user queries.
- Engagement rate: Measuring the level of user engagement with the chatbot, such as the number of interactions per session.
- Conversion rate: Tracking the percentage of users who complete a specific goal or action after interacting with the chatbot.
Metrics for evaluating user engagement
User engagement metrics are crucial in determining the effectiveness of an AI chatbot. These metrics focus on how users interact with the chatbot and can provide valuable insights into user satisfaction and overall performance.
Time spent interacting with the chatbot
One key metric for evaluating user engagement is the amount of time users spend interacting with the chatbot. Longer interaction times typically indicate that users find the chatbot helpful and engaging. Monitoring this metric can help identify areas where the chatbot can be improved to keep users engaged for longer periods.
Number of interactions per session
Another important metric is the number of interactions users have with the chatbot in a single session. A higher number of interactions can indicate that users are actively engaging with the chatbot and seeking information or assistance. By tracking this metric, chatbot developers can tailor the chatbot’s responses to provide more relevant and valuable information to users.
Retention rate
The retention rate measures how many users return to interact with the chatbot after their initial session. A high retention rate suggests that users find the chatbot valuable and continue to engage with it over time. To improve retention rates, chatbot developers can implement features that encourage users to return, such as personalized recommendations or proactive messaging.
Feedback and sentiment analysis
Collecting feedback from users and analyzing their sentiment towards the chatbot is another important user engagement metric. Positive feedback and sentiment indicate that users are satisfied with the chatbot’s performance, while negative feedback can highlight areas for improvement. By actively seeking feedback and monitoring sentiment, chatbot developers can make continuous improvements to enhance user engagement.
Personalization and customization options
Offering personalized and customizable options within the chatbot can also improve user engagement. By allowing users to tailor their interactions based on their preferences and needs, chatbots can provide a more personalized and engaging experience. Features such as personalized recommendations, customizable settings, and interactive elements can all contribute to higher levels of user engagement.
Metrics for assessing conversational quality
Conversational quality metrics are essential for evaluating the effectiveness of interactions between users and chatbots. These metrics provide insights into how well the chatbot can engage in meaningful conversations and meet user needs.
When it comes to assessing conversational quality, there are several key metrics to consider. These metrics help measure the chatbot’s ability to understand user inputs, provide relevant responses, and maintain a natural flow of conversation. By analyzing these metrics, businesses can identify areas for improvement and enhance the overall user experience.
Response Accuracy
Response accuracy is a crucial metric that evaluates how well the chatbot understands user queries and provides relevant information. High response accuracy indicates that the chatbot can effectively interpret user inputs and deliver accurate responses in a timely manner.
Response Relevance
Response relevance measures the chatbot’s ability to provide meaningful and contextually appropriate responses to user queries. This metric assesses whether the chatbot can understand the user’s intent and deliver responses that align with the conversation flow.
Conversation Flow
Conversation flow evaluates the coherence and continuity of interactions between the user and the chatbot. A smooth conversation flow ensures that the chatbot can maintain a natural dialogue structure and transition seamlessly between topics.
User Satisfaction
User satisfaction is a key metric that reflects the overall quality of the conversation from the user’s perspective. By measuring user satisfaction through feedback and ratings, businesses can gauge how well the chatbot met user expectations and provided a positive experience.
By focusing on these conversational quality metrics, businesses can enhance the effectiveness of their chatbots and improve the overall user experience. Strategies for enhancing conversational quality include continuously training the chatbot on new data, refining response algorithms, and incorporating user feedback to make informed improvements.
Common Queries
How do AI chatbot performance metrics impact user experience?
AI chatbot performance metrics provide valuable data insights that help businesses understand user interactions, preferences, and pain points. By analyzing these metrics, companies can tailor their chatbot strategies to enhance user experience and drive engagement.
What are some common examples of key performance indicators (KPIs) for chatbots?
Common KPIs for chatbots include response time, resolution rate, user satisfaction, conversation completion rate, and retention rate. These metrics help businesses track the effectiveness of their chatbots and make informed decisions for optimization.